Artificial intelligence steps up on the flight deck and in the back office.
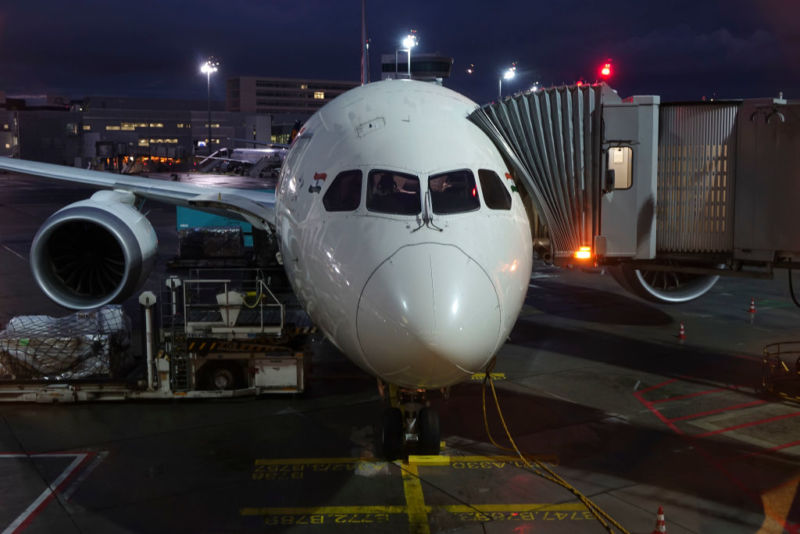
Boeing 787 Dreamliner.
Welcome to Ars UNITE, our week-long virtual conference on the ways that innovation brings unusual pairings together. Each day this week from Wednesday through Friday, we're bringing you a pair of stories about facing the future. Today's focus is on AI in transportation-buckle up!
Ask anyone what they think of when the words "artificial intelligence" and aviation are combined, and it's likely the first things they'll mention are drones. But autonomous aircraft are only a fraction of the impact that advances in machine learning and other artificial intelligence (AI) technologies will have in aviation-the technologies' reach could encompass nearly every aspect of the industry. Aircraft manufacturers and airlines are investing significant resources in AI technologies in applications that span from the flightdeck to the customer's experience.
Automated systems have been part of commercial aviation for years. Thanks to the adoption of "fly-by-wire" controls and automated flight systems, machine learning and AI technology are moving into a crew-member role in the cockpit. Rather than simply reducing the workload on pilots, these systems are on the verge of becoming what amounts to another co-pilot. For example, systems originally developed for unmanned aerial vehicle (UAV) safety-such as Automatic Dependent Surveillance Broadcast (ADS-B) for traffic situational awareness-have migrated into manned aircraft cockpits. And emerging systems like the Maneuvering Characteristics Augmentation System (MCAS) are being developed to increase safety when there's a need to compensate for aircraft handling characteristics. They use sensor data to adjust the control surfaces of an aircraft automatically, based on flight conditions.
But machine-learning systems are only as good as the data they get. There is inherent risk in handing off more of what humans do in a high-risk environment to ML or AI that few people understand. While the final investigation of the recent crash of Lion Air 610 is still underway, the details revealed so far are a strong warning of the risks of handing off too much control to autonomous systems. While catastrophic aviation accidents seldom happen as a result of a single mistake (and this was no exception), the MCAS sensors failed, maintenance failed to fully correct the issue, and the pilots had not been fully trained and informed on the function and use of the MCAS.
The lesson, reinforced at a tragic cost of 189 lives, is that the aviation industry will have to fold data quality and the care and feeding of ML and AI systems into the safety culture that commercial aviation is already renowned for. As machine learning and AI transform the role of pilots, those technologies need to be as thoroughly tested as their human counterparts and deemed at least as competent.
Beyond the auto-pilot
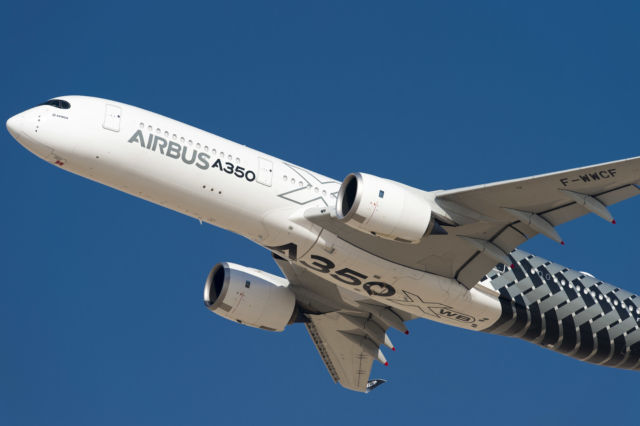
The Airbus A350 XWB aircraft, shown here during the Dubai Airshow in 2015, has more than 50,000 sensors collecting flight and performance data totaling over 2.5TB a day.
Major aircraft manufacturers such as Airbus are already phasing in AI. According to Airbus Vice President for AI Adam Bonnifield, the company has been working on these technologies for a long time. "Airbus is not that unfamiliar with these technologies because of our background in aviation and building systems that essentially solve some problems in autonomy," he told Ars.
There's plenty of data to tap regarding machine learning aboard the modern airliner: the A350 XWB, Airbus' twin-engine wide-body aircraft introduced in 2015, has some 50,000 sensors and collects 2.5 terabytes of data daily. And AI can make use of that data in a number of ways. Airbus is working on projects that reduce the cognitive load (and the resulting cognitive fatigue) on pilots, as well as the number of pilots required to be at the controls. This means the crew can spend more time handling the overall strategy and mission of a flight and less time dealing with all the small sub-problems of piloting an aircraft.
Bonnifield explained that, while many people view autonomy in aircraft as "a binary"-either an airplane is autonomous or it isn't-he feels differently. "It's more of a spectrum," he said, "where we take some of the small problems of flying a plane and try to use AI to solve them."
One example of this is an option available on Airbus aircraft called runway overrun protection. ROPS is software that calculates aircraft approach speed and weight, and it compares the resulting physics model with the published runway length and current local weather on approach. If it detects an unsafe situation, it broadcasts the message "Runway too short!" ROPS also calculates optimal approach glide-slopes, or trajectories, for a landing approach, and it helps with taxiing, takeoff, and other aspects of flight.
Another area of AI focus at Airbus is building autonomous vehicles and air taxis designed to transport people inside urban areas. And AI could potentially be used in a passenger plane when the pilots are rendered unconscious from a fall in cabin pressure. It can add up factors and make better decisions faster under high-pressure situations than humans given the right data, creating a potential increase in safety.
Simplifying communications
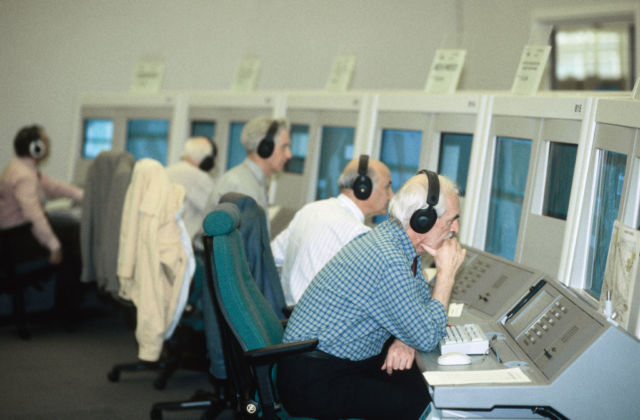
Air traffic controllers at the NATS London Area Control Centre LACC in Swanwick, UK. Heavily accented English over noisy communications channels is a real test of AI voice recognition.
Air Traffic Control (ATC) communications is a critical aspect of all flights. In the European airspace, much conversation happens in heavily accented English, making it difficult for pilots and controllers to understand each other. Pilots need to listen for their tail/flight number to be called for clearances, directional instructions, and traffic alerts, often under challenging instrument meteorological conditions (IMC) when they can't see out of the cockpit. Airbus directed AI at this problem as part of a public contest in the company's AI Gym-a program in which Airbus seeks outside partners to assist in developing breakthrough AI systems.
Cleaning up air traffic conversations is difficult for machine-learning algorithms to parse, because ATC audio is noisy, and the conversation is rapid-fire and full of what Airbus described as "domain-specific vocabulary." The goal of AI Gym was to provide full transcription of ATC audio, as well as extract aircraft call signs from audio for conversation tracking and alerting.
"We opened it up to a broad community of different businesses, consulting firms, startups, and research groups to collaborate with us," said Bonnifield. The competition closed in October 2018, and Airbus has already begun work to convert the results into a product.
The AI Gym program has allowed Airbus to attack a number of other potential uses for AI by leveraging outside expertise. "We have these interesting problems and use cases that are largely unexplored and unsolved," Bonnifield said. "Partly because of the fact that the space is so new, we're living at this very immature inflection point of the technology where there's a lot of experimentation happening and even some terrific open source technology."
Through the program, Airbus is working with "all the usual suspects," Bonnifield said; the projects are all performed under non-disclosure agreements. The anonymity of the NDA can be a good thing, he suggested, because not every effort is successful-and failures aren't advertised. While "the usual suspects" in machine learning might often be expected to be the companies to come up with the highest-performing solution, Bonnifield said he discovered that most of the time the best solutions come from tiny startups.
Often, research teams with only a few people are able to produce the best solution. Bonnifield said he believed this is probably unique to the AI space. Airbus' big challenge is how to bring these small teams at the tip of the innovation spear along and give them an easy way to collaborate. That has required Airbus to change the way it works with outsiders. "Some of the startups have never done an RFP [Request for Proposals response] before," Bonnifield explained.
Getting to business
When it comes to flight-safety issues, airlines rely heavily on their equipment manufacturers (such as Airbus and Boeing). But airlines aren't just counting on AI to assist on the flight deck. Machine learning and AI are being called upon in the back office to help airlines in their battle to streamline ground operations and to create the best customer experience by making travel as painless and seamless as possible.
United Airlines Vice President of Digital Products and Analytics Praveen Sharma said that United is investing in all available new technology to use machine learning with the backend data it gathers from customers, maintenance logs, employee duty logs, and in-flight progressive data to improve all aspects of its business.
In September, United and Palantir Technologies announced a long-term relationship to deploy Palantir Foundry to accelerate enterprise-wide data initiatives across a range of critical business units as the airline's central platform. According to Sharma, "One challenge... we are trying to solve is how to bring this vast amount of data from various parts of the company on different platforms onto a single platform... [that] we can leverage for our machine learning and AI model." The two companies have been working on a wide range of projects for the past year to do this.
Palantir partnered with Airbus to create Skywise, an aviation data-analytics platform that Airbus provides to smaller airlines as a subscription service that would include tools to help reduce unplanned maintenance on aircraft. GE has also tried to turn aircraft sensor data into a machine-learning-based service to drive predictive maintenance of the company's jet engines.
United and its regional carrier, United Express, operate about 4,600 flights a day to 357 airports across five continents. Last year, the companies operated more than 1.6 million flights carrying more than 148 million customers. When unforeseen maintenance issues do occur or other operational issues get in the way, United is using machine learning to help swap out aircraft. This isn't as simple as one might expect; the system must take into account all of the variables required for assigning a crew (such as rest time and appropriate crew aircraft certifications), aircraft fuel and operations limitations, and aircraft seating capacity. "These are complicated decisions that often must be calculated and decided in a 25-minute timeframe based on the limited amount of data available at that time," Sharma explained.
Beyond maintenance
But United's use of machine learning and AI goes far beyond managing maintenance and aircraft schedules. It also taps into customer data. Using the data gleaned from each passenger interaction, United is applying AI and machine learning to streamline its customers' experience based on their data-and tuning offers to match their profiles.
United's machine-learning algorithms take 150 different customer and flight data points and, in real time, decide which particular product to put in front of a customer at the purchase or check-in point. The engine takes into account things like passengers' previous purchases, preferences, destinations, and activities. Customers' interactions move through United's real-time decision engine, up and running since 2014, which gives them various product options to improve their travel experience. Options include flight choice, seat upgrades, mileage purchase, or the ability to jump to the front of the line with Priority Access.
To drive what gets offered up to each customer, Sharma said that United uses a prediction model based on a Bayesian inference model. "It decides not just what offer to give," Sharma explained, "but what image to put in front of the customer and what tagline to use."
Sharma said that the application of machine learning is paying off. Based on measurements collected by United, customers aren't having to hunt for things they want to purchase or for desired experiences.
Other airlines are embracing AI in other forms to take the pain out of travel (and to reduce the workload on airline employees). Facial-recognition technology is now showing up on terminal kiosks to help speed check-in at the airport.
Most facial-recognition algorithms are based on deep learning, which is part of machine learning. Delta Airlines is the first to deploy this process, speeding up passengers' time to gate by almost 10 minutes, according to the airline's estimates. The system, used currently for check-in and baggage check on international flights, leverages passengers' passport photos. Delta expects to expand operations to domestic flights next year.
Preventing future disasters
Perhaps one of the most important uses of AI-based analytics, however, may be in identifying risks to the safety of aircraft before a disaster-such as the crash of Lion Air Flight 610, when a failure of the automated control system on a prior flight may have signaled a major safety issue. NASA Ames Research Center in Silicon Valley is heavily involved in aviation-related AI, and one of NASA's projects there is focused on identifying "anomalous operations" within data from commercial aviation-events that could be precursors to potentially bigger problems.
This is a primary area of research for Nikunj Oza, a computer scientist and leader of the data sciences group within NASA Ames' intelligent systems division known as Code TI. Because commercial aviation's safety record is so good-much better than driving, for example-it's much more difficult to identify those few cases where there's an anomaly that might represent a safety issue.
NASA has done some initial development of algorithms related to anomaly detection and incident precursor identification, and it has started the process for gathering feedback from experts in the field. The agency is currently developing a system for use in safety analysis of aircraft data-in particular, for FAA's analytics partner Mitre, the federally funded research and development center. Mitre runs a program called Aviation Safety Information Analysis and Sharing (ASIAS), a data consortium that shares safety data among NASA, the Federal Aviation Administration, the National Transportation Safety Board, aircraft manufacturers, and more than 50 airlines. The airlines upload some subsets of their flight-recorded data to Mitre, which performs analysis and provides feedback on potential problems. (The data is shared confidentially by the airlines.)
The hope for the analytics being developed at Ames is that the AI can discover patterns of anomalies in flight data that could be indicative of a systematic problem with aircraft. "You'd like to find that as soon as possible and come up with some kind of a mitigation to prevent it happening again," Oza explained. He said that, so far, rather than AI replacing humans outright in aviation, AI and human experts have proven to be complementary-a partnership that can save human lives.
Ingen kommentarer:
Legg inn en kommentar
Merk: Bare medlemmer av denne bloggen kan legge inn en kommentar.